SLAM, which stands for “Simultaneous Localization and Mapping,” is a crucial technology used in autonomous systems such as mobile robots and unmanned vehicles. Its purpose is to enable robots to localize themselves in real-time in unknown environments while simultaneously constructing a map without prior knowledge of the environment. SLAM plays a significant role in autonomous navigation and environment perception.
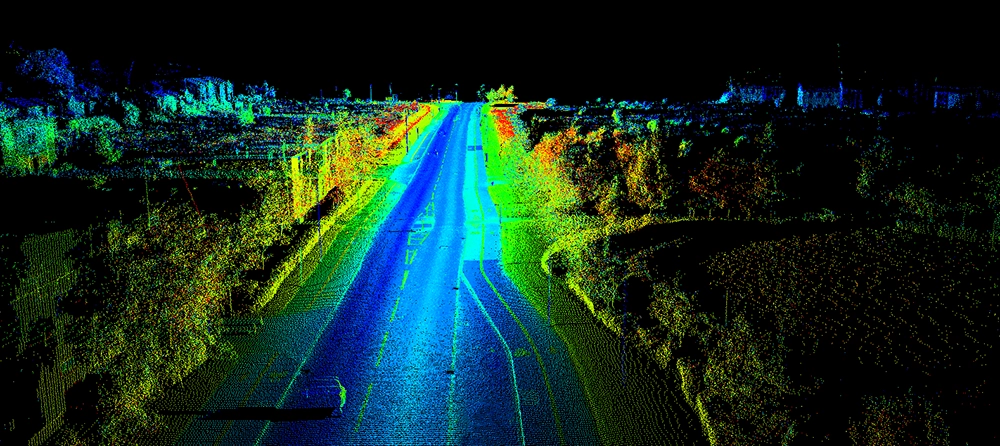
Implementation Principle: The implementation of SLAM relies on sensor data fusion, probabilistic filtering, and optimization algorithms. Common sensors used include LiDAR, cameras, inertial measurement units (IMUs), and odometry.
- Data Fusion: The robot gathers environmental information from multiple sensors, such as LiDAR for measuring obstacles and cameras for visual information. SLAM algorithms fuse this sensor data to obtain a more accurate and comprehensive representation of the environment.
- Front-end Processing: In the front-end processing stage, SLAM algorithms detect and match feature points, such as corners and lines, to track their motion and estimate the robot’s pose (position and orientation).
- Back-end Optimization: SLAM algorithms use probabilistic filtering or graph optimization techniques to optimize the robot’s trajectory and the constructed map. These optimization algorithms help reduce sensor measurement errors and motion estimation accumulation errors, leading to more accurate maps and robot pose estimates.
Applications in the Surveying and Mapping Industry: SLAM technology finds extensive applications in the surveying and mapping industry, particularly in indoor environments or situations where traditional surveying methods are impractical or cost-prohibitive. It provides an efficient and economical solution for mapping tasks.
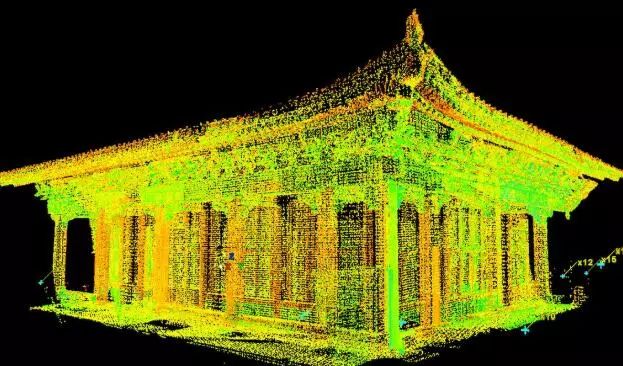
- Building and Indoor Mapping: SLAM is used for mapping and modeling indoor spaces of buildings. Mobile robots or drones equipped with LiDAR and cameras can autonomously explore and map the interior of unknown environments.
- SLAM technology has undergone several stages of development in the field of building and indoor mapping. With advancements in sensor technology and algorithms, SLAM has become more powerful and widely used in these applications. Below are the main development stages of SLAM in building and indoor mapping:
- Initial Stage: In the early days, SLAM technology relied on relatively simple sensors, such as laser rangefinders and encoders. These sensors provided limited environmental information, and the algorithms were relatively straightforward. However, due to sensor accuracy and algorithm limitations, SLAM faced challenges in positioning and mapping in large-scale and complex environments.
- Diverse Sensors: With the advancement of sensor technology, modern SLAM systems use a variety of sensors, including LiDAR, RGB-D cameras, inertial measurement units (IMUs), and cameras. The fusion of multiple sensors allows SLAM to obtain environmental information from different perspectives, enhancing the accuracy and stability of localization and mapping.
- Rise of Visual SLAM: Visual SLAM, which utilizes cameras to capture environmental information, emerged as an essential branch in the SLAM field. Compared to traditional LiDAR-based SLAM, visual SLAM is relatively cost-effective and easy to deploy. Additionally, visual SLAM provides rich visual information, such as feature points and scene structures, making it advantageous for indoor environments. Moreover, the development of deep learning has improved the real-time performance and accuracy of visual SLAM.
- Improved Real-Time Performance and Accuracy: With the improvement of computational hardware and algorithm optimization, SLAM technology has significantly enhanced its real-time performance and accuracy. Modern SLAM systems can achieve real-time localization and mapping in a short period, providing high-precision localization and mapping information in most scenarios.
- Applications in Building and Indoor Navigation: SLAM technology’s application in building and indoor mapping has expanded into navigation and positioning domains. Robots or drones based on SLAM can autonomously navigate indoor environments, create floor plans, and help users quickly find target locations, improving indoor navigation efficiency and accuracy.
- Integration with Building Information Modeling (BIM): The integration of SLAM technology with Building Information Modeling (BIM) has made indoor mapping more comprehensive and accurate. SLAM can be used to rapidly create initial BIM models, while BIM information aids SLAM in environment feature recognition and map construction, forming a mutually complementary closed-loop system.
- Autonomous Vehicles: In autonomous driving, SLAM technology is essential for achieving self-localization and real-time map updates. By fusing data from LiDAR, cameras, and other sensors, vehicles can perceive the surrounding environment and plan optimal paths.
- Underground Mines and Tunnel Mapping: In hazardous or hard-to-reach underground environments, SLAM can be used for autonomous exploration and mapping by robots or drones, aiding in better understanding the environment and planning operations.
- Environmental Monitoring: SLAM can be applied to monitor and map the impacts of natural disasters such as floods, fires, and earthquakes. Drones or mobile robots can enter disaster areas for environmental exploration and real-time data collection.